Prerequisites of linear algebra for machine learning
Just about everyone has watched animated movies such as Frozen or Big Hero 6 or has at least heard about 3D computer games. It seems more fun to enjoy the movies and games rather than reading a Linear Algebra book. But it is because of linear algebra that we are able to watch a character move on the screen. Linear Algebra is the motivation of our new digital world.
Through this article, we will learn matrix arithmetic and learn how to use numpy to carry out these operations in python.
Why we need Linear Algebra for Machine Learning?
Machine Learning deals with the handling of enormous data sets. And an effective way to represent this data is in the form of 2D arrays or rectangular blocks in which each row represents a sample or a complete record and a column represents a feature or an attribute. It is natural to think of the array as a matrix and each column (attribute) as a vector.
Python and Linear Algebra
Numpy is a library used for scientific computing in Python. It provides multidimensional arrays and tools for working with these arrays.
Matrices
A matrix is a rectangular array of numbers (usually real) represented in forms of rows and columns. For example,
\(\begin{bmatrix}1 & 2 & 3\\100 & -3 & 1.15\end{bmatrix}\)
The dimension of a matrix is written as number of rows \(\times\) number of columns. So the dimension of above matrix is 2[math]\times[/math]3. A general m[math]\times[/math]n matrix is of the form
Hire top tech talent with our recruitment platform
Access Free Demo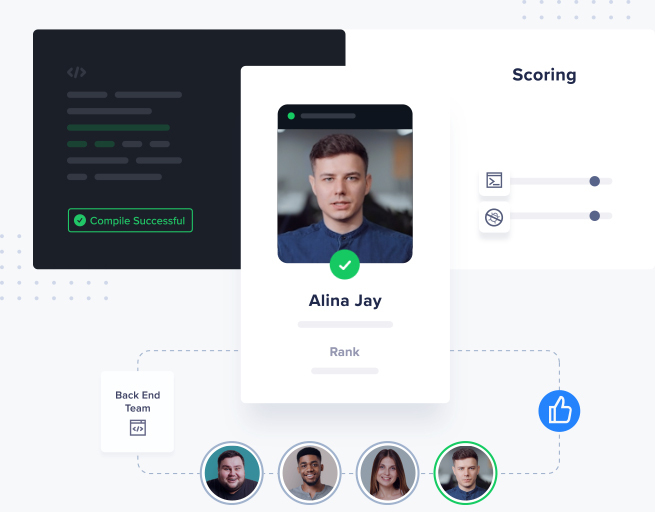
A = \(\left[\begin{array}{cccc}a_{11} & a_{12} & \dots & a_{1n}\\a_{21} & a_{22} & \dots & a_{2n}\\\dots&\dots&\dots&\dots\\a_{m1} & a_{m2} & \dots & a_{mn}\end{array}\right]=\left(a_{ij}\right)_{m\times n}\)
.array()
It helps define multidimensional arrays.
>>>import numpy as np >>>A=np.array([[1,2,2],[3,2,1]]) >>>print A [[1 2 2] [3 2 1]]
matrix_name.shape
Returns (number of rows, number of columns)
>>>import numpy as np >>>A=np.array([[1,2,2],[3,2,1]]) >>>print A.shape (2,3)
Identity Matrix
An identity matrix, I of dimension n[math]\times[/math]n is the matrix in which all the elements on principal diagonal are 1 and the rest are 0.
.eye(n)
Returns n[math]\times[/math]n Identity Matrix
>>>import numpy as np >>>A=np.eye(3) [[1 0 0] [0 1 0] [0 0 1]]
Square Matrix
A matrix is called a square matrix if the number of rows is equal to the number of columns. An identity matrix is always a square matrix.
Matrix Operations
Matrices are mathematical objects on which we can perform multiple operations like addition, multiplication, etc.
-
Addition
Addition of matrices is only defined for matrices of the same dimension as addition is done entry wise, i.e., for two matrices
A = \(\left(a_{ij}\right)_{m\times n}\) and B = \(\left(b_{ij}\right)_{m\times n} \)
A+B = \(\left(a_{ij}+b_{ij}\right)_{m\times n}\)
Suppose Amy, Sheldon, Leonard, Raj, and Alex have 3000 $ each to invest in Mutual Funds of Reliance and Airtel in September 2016.
Name Investment in Reliance MF Investment in Airtel MF Amy 1500 1500 Sheldon 2300 700 Leonard 0 3000 Raj 1000 2000 Alex 1900 1100 All of them also made investments in August 2016 as follows-
Name Investment in Reliance MF Investment in Airtel MF Amy 1000 2000 Sheldon 2100 900 Leonard 3000 0 Raj 1200 1800 Alex 1400 1600 Now if we have
A =\(\begin{bmatrix}1500 & 1500\\2300&700\\0&3000\\1000&2000\\1900&1100\end{bmatrix}\) and B = \(\begin{bmatrix}1000 &2000\\2100&900\\3000&0\\1200&1800\\1400&1600\\\end{bmatrix}\)
then A+B = C = \( \begin{bmatrix}2500 & 3500\\4400 & 1600\\3000&3000\\2200&3800\\3300&2700\end{bmatrix}\)Here C signifies the total investments made by Amy, Sheldon, Leonard, Raj and Alex in August and September. For instance, the total investment made by Leonard in Airtel Mutual Fund is \(c_{32}=3000\)$.
.add(Matrix 1, Matrix 2)
Returns Matrix 1 + Matrix 2
>>>import numpy as np >>>A=np.array([[1,2,2],[2,3,4]]) >>>B=np.array([[5,7,3],[3,-3,0]]) >>>C=np.add(A,B) >>>print C [[6,9,6] [8,4,3]]
Some properties of addition of matrices are as follows-
Associativity: \((A+B)+C = A+(B+C)\)
Commutativity: \(A+B = B+A\) -
Transposition
Transpose of a \(m\times n\) matrix, A is the matrix obtained by interchanging rows and columns of A. It is denoted by [math]A^\mathsf{T}[/math]. Clearly, [math]A^\mathsf{T}[/math] is a \(n\times m\) matrix.
If A = \(\begin{bmatrix}1 & 2 & 3\\100 & -3 & 1.15\end{bmatrix}\) then[math] A^\mathsf{T}[/math]=\(\begin{bmatrix}1 & 100\\2 & -3 \\3& 1.15\end{bmatrix}\)
.transpose(A)
Returns [math] A^\mathsf{T}[/math]
>>>import numpy as np >>>A=np.array([[1,2,2],[2,3,4]]) >>>C=np.transpose(A) >>>print C [[1,2] [2,3] [2,4]]
Transposing will be useful in the multiplication of vectors.
-
Multiplication
Multiplication of any two arbitrary matrices is not possible. For two matrices to be compatible for multiplication, the number of columns in the first matrix should be equal to the number of rows in the second matrix.
That is, for two matrices A and B, AB exists only if the dimension of A is \(m\times n\) and the dimension of B is \(n\times q\). AB has dimension \(m\times q\).
To multiply two matrices, we take the dot product of rows and columns, i.e., say, A =\(\begin{bmatrix}1&2&3\\4&5&6\end{bmatrix}\) and B =\(\begin{bmatrix}1&5&1\\3&2&0\\-1&1&3\end{bmatrix}\)This might look a little more abstract as compared to the addition of matrices. Let’s look at the intuition behind this.
Consider the example we used above. Now, if Reliance MF gives a dividend of 10% in the month of September and Airtel had a loss and charges an extra 2%, what is the net gain/loss made by, say, Sheldon in the month of September?
Net Gain/Loss= \(0.1\times2300\) – \(0.02\times700=216\)
Let’s look at this in terms of matrices-New Reliance Mutual Fund \(\begin{bmatrix}1.1 & 0\end{bmatrix}\)
New Airtel Mutual Fund \( \begin{bmatrix}0 & .98\end{bmatrix}\)
Net Profit \( \begin{bmatrix}0.1 & -0.02\end{bmatrix}\)To calculate net gain by each investor for both the mutual funds we calculate
\(\begin{bmatrix}1.1 & 0\\0&0.98\\0.1&-0.02\end{bmatrix}\begin{bmatrix}1500 & 1500\\2300&700\\0&3000\\1000&2000\\1900&1100\end{bmatrix}^\mathsf{T}=\begin{bmatrix}1.1 & 0\\0&0.98\\0.1&-0.02\end{bmatrix}\begin{bmatrix}1500&2300&0&1000&1900\\1500&700&3000&2000&1100\end{bmatrix}\)
\(=\begin{bmatrix}1650 & 2530 & 0 & 1100 & 2090\\1470 & 686 & 2940 & 1960 & 1078\\120 & 216 & -60 & 60 & 168\end{bmatrix}\)So the above calculated matrix represents-
Amy Sheldon Leonard Raj Alex Current Value Of Reliance MF 1650 2530 0 1100 2090 Current Value Of Airtel MF 1470 686 2940 1960 1078 Net Gain 120 216 -60 60 168 Following is a list of properties of multiplication of matrices-
Associativity: \((A.B).C=A.(B.C)\)
Non-commutativity: \(A.B \neq B.A\)
Transposition rule: \((A.B)^T=B^{T}.A^{T}\)
\(A.I=I.A=A\) -
Inversion
We define the inverse of real number, [math]r[/math] as an other real number [math]r^{-1}[/math] such that [math]r.r^{-1}=1[/math]. For instance the inverse of 2 is \(\frac{1}{2}=0.5\) and that of 3 is \(\frac{1}{3}\). Not all real numbers have inverses like 0 because \(\frac{1}{0}\) is an undefined quantity.
Similarly, we can define the inverse of a matrix A as \(A^{-1}\) such that \( A.A^{-1}=I\). Again, not all matrices have inverses. Rather only a category of square matrices is invertible. For instance the matrix [math]A=\begin{bmatrix}2&3\\4&5\end{bmatrix}[/math] has inverse[math]A^{-1}=\begin{bmatrix}-2.5 &1.5\\2&-1\end{bmatrix}[/math].linalg.inv(Matrix name)
It returns the inverse of a specified array.
>>> import numpy as np >>> A=np.array([[2,3],[4,5]]) >>> B=np.linalg.inv(A) >>> print B [[-2.5 1.0] [2.0 -1.0]]
Vectors
A vector is an n-tuple of values. Conventionally, it is represented as a \(n\times1\) matrix but can also be written as a \(1\times n\) matrix. The dimension of a n-tuple vector is n and n can be any positive integer from 1 to infinity. The general form of a n dimensional vector is
[math]v=\begin{pmatrix}v_1\\v_2\\\vdots \\v_n\end{pmatrix}[/math]
Vector arithmetic is similar to matrix arithmetic.
-
Addition
[math]v+w=\begin{pmatrix}v_1\\v_2\\\vdots \\v_n\end{pmatrix}+\begin{pmatrix}w_1\\w_2\\\vdots \\w_n\end{pmatrix}
=\begin{pmatrix}v_1\mbox{ + }w_1\\v_2\mbox{ + }w_2\\\vdots \\v_n\mbox{ + }w_n\end{pmatrix}[/math] -
Multiplication
Multiplication of two vectors is similar to the dot product of two vectors which returns a scalar.
[math]v.w=vw^{T}=\begin{pmatrix}v_1\\v_2\\\vdots\\v_n\end{pmatrix}\begin{pmatrix}w_1&w_2
&\ldots&w_n\end{pmatrix}=\begin{pmatrix}v_1w_1+v_2w_2+\ldots+v_nw_n\end{pmatrix}[/math].dot(v,w)
Returns \(vw^{T}\)
>>> import numpy as np >>> A=np.array([[1,2,3]]) >>> B=np.array([[5,6,7]]) >>> C=np.dot(A,B) >>> print C 38.0
In the next article we will see the application of matrix arithmetic to principal component analysis which deals with identifying patterns in data.

Get advanced recruiting insights delivered every month
Related reads
How To Conduct A Recruitment SWOT Analysis?
A SWOT analysis is a business strategy to assess the Strengths, Weaknesses, Opportunities and Threats of a system. The exercise helps teams evaluate…
How to Build a Recruitment Pipeline for Seasonal Hiring
Seasonal hiring can be a daunting task, whether it is peak accounting season for finance companies or the time for a product launch,…
Best Practices for Writing Inclusive Job Descriptions
The hiring landscape has seen a paradigm shift in terms of diversity in people, talent, skills and above all, emphasis on emotional intelligence…
Benefits Of AI-Powered Job Descriptions
The introduction of AI in recruitment has revolutionized how hiring workflows are designed. It paved the way for new-age recruiters to enhance the…
Benefits of Recruitment Process Outsourcing (RPO)
Today’s era has seen a steep increase in the use of technology in hiring and outsourcing the hiring process. To keep up with…
AI-Enhanced Job Matching: Finding the Perfect Fit
Today’s job landscape has become increasingly competitive for both job seekers and recruiters. One of the main challenges recruiters face is finding the…
