Unlock skill-first hiring with HackerEarth today
Learn more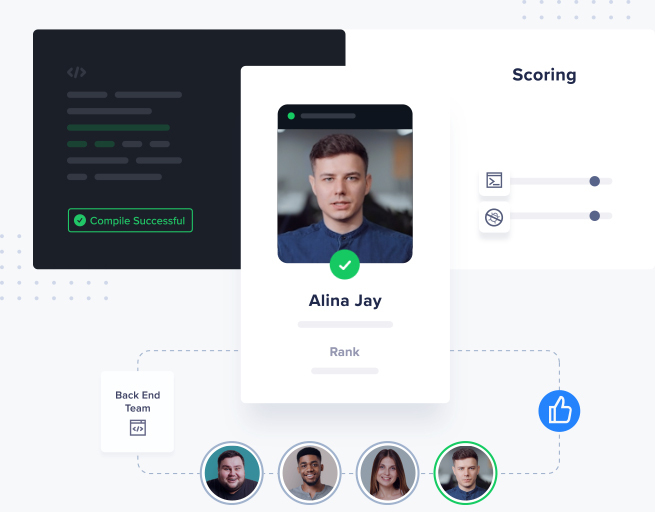
How to solve nondeterministic polynomial (NP) challenge problems in programming contests
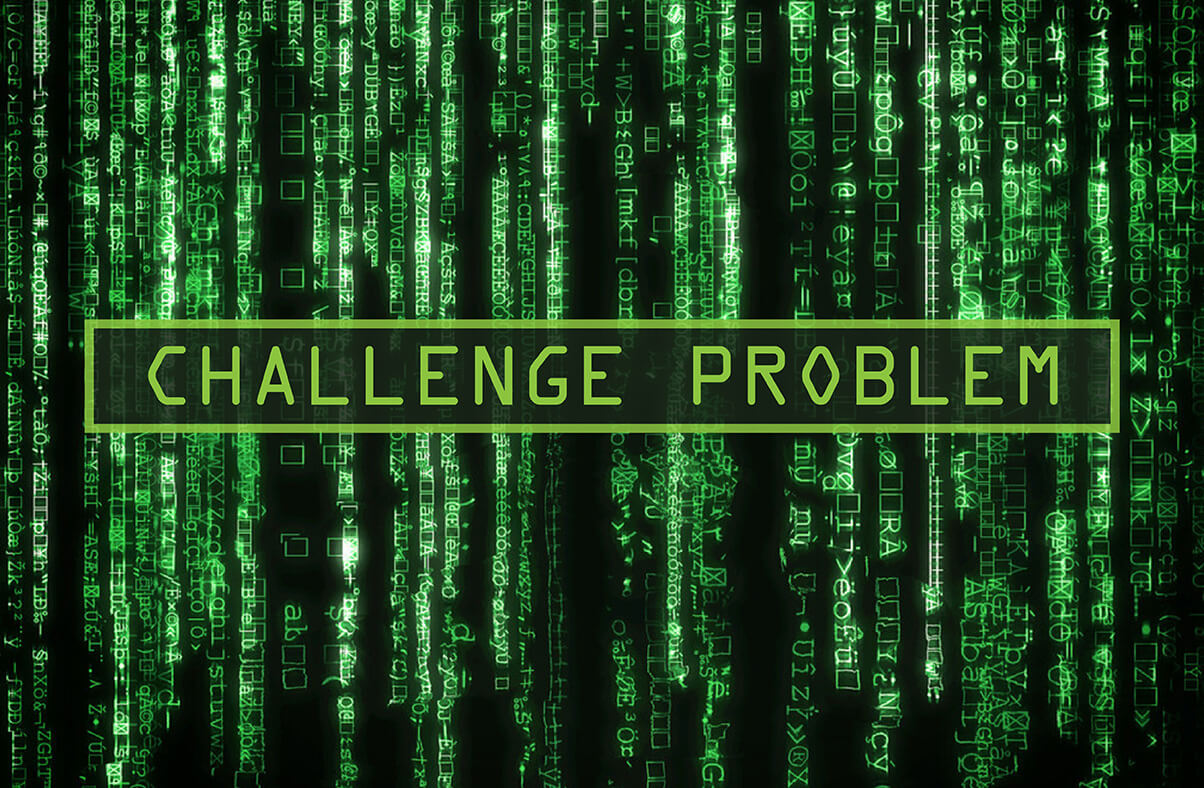
In this article, we talk about what Challenge problems are and how to solve them. I find them the most attractive questions in a long contest.
However, students new to competitive programming often avoid them because they seem weird at first. Let’s try and change that perception.
What are Challenge problems?
A challenge problem in a programming contest uses NP (Nondeterministic Polynomial) problems to test a candidate.
With no perfect answer, candidates are tested on how good they are at finding approximate solutions.
This is why the evaluation is score based.
The top scorer is given a score of 100, while the others are given relative scores.
Usually, problem statements are simple and allow candidates to find interesting nuances while solving.
Because of the generality of the problem, candidates can choose numerous approaches to optimize their score.
These include Number Theory, Graphs, Data Science, etc…
Some problems require prior knowledge of algorithms, but the best challenge problems are those which have simple explanations and lots of scope for improvement.
Such problems allow beginners to try and test their approximate solutions while making sure that the experienced players are tested too.
How do we solve this:
Watch this brilliant video by MIT professor Patrick Wilson, who solves a problem (at 42:30) and amazing trick he puts into it
To summarize the procedure for the problem discussed by the professor, here are the steps –
- Definition
Does the problem reduce to Knapsack? Subset sum? Something else? - Representation
Should the main data structure be an array, a list or a tree? - Approach
Dynamic Programming? Graph Algorithm? etc… - Algorithm
DFS / Sorting / Edit Distance –> Simulated Annealing / Genetic Algorithm - Experiment
A key point is that drawing and discussing ideas are far more important than jumping into code. The other super important thing is you can’t follow this process in just one shot.
It has to be repeated, with each iteration improving your solution.
First, you need a base solution. Choose a simple, clear-cut algorithm (like the first three mentioned above in point 4).
This is the foundation, setting a minimum guarantee to your score.
Invest your time in this, because a weak base solution will directly result in a poor score.
You now need to tweak the best solution you have, updating if you get something better than the current best.
Hill climbing, Simulated Annealing, or any Evolutionary Algorithm will help you here.
Most of your time after this will be spent improving the parameters of the algorithm and improving the time complexity.
The time required to solve them
They can’t be solved in half a day, to be practical. Unless you are looking for an okay score and minimum effort, be prepared to invest significantly.
Also, beware of the law of diminishing returns. In general, the more time you invest in these problems, the smaller the improvements you will see.
The first few hours will give you interesting solutions => perhaps a score of 75–85; the next few days might take you to 95–100. And staying there takes tons of effort.
The primary reason why these problems are difficult is that we have to customize and optimize our solutions every time.
You can create a general framework for Simulated Annealing to help in the coding phase. However, the “representation” part will change every time.
Up to which ‘t’ point do I keep optimizing
Until you feel that there is very little progress being made. To be practical, the other problems will give you many more points for the same amount of effort.
When you hit problems beyond your league, come back to improving on the challenge problem solution.
Remember that the people at the top have access to the same resources as you do. There is no reason why they should get a better score than you.
When should I start with this problem
This problem will stay at the back of your mind throughout the contest. So, it is better to start a little late.
More often than not, the problems within our league are solved within the first 80% of the contest’s duration.
The challenge problem can be started then.
Final tips and tricks:
- Do not jump into meta-heuristics (Genetic Algorithms, Simulated Annealing) without creating a strong base solution.
- When optimizing, try to keep the code clean for later.
- Your nested loops need the most attention because that’s where you get the maximum gains.
- Greed is good. Keep your heuristics simple and easy to change.
- If some idea feels too complicated, it is. Save it for the next contest, after reading a little more and being confident about it.
Practice your learning at one of the challenges at HackerEarth Challenges

Get advanced recruiting insights delivered every month
Related reads
Top 10 HR Competencies to Build a Strong HR Department: A Comprehensive Guide
Introduction In today’s dynamic workplaces, a strong HR department is no longer a luxury – it’s a necessity. HR professionals play a crucial…
8 Steps for Conducting a Job Tasks Analysis: A Complete Guide
Job task analysis is a crucial process for understanding the specific duties and skills required for a particular role. By incorporating insights from…
Top 8 Sourcing Tools for Recruiters: A Comprehensive Guide
In today’s competitive talent landscape, attracting top candidates requires going beyond traditional job board postings. This is where effective sourcing tools comes into…
The 12 Most Effective Employee Selection Methods: A Comprehensive Guide
Finding the perfect fit for your team can feel like searching for a unicorn. But fret not, fellow recruiters! Here’s where employee selection…
12 Important Recruiting Metrics You Should Know
Recruitment forms a strong foundation to build an effective team. However, do you know if your recruitment strategy is working or not? This…
7 Modern Performance Appraisal Methods to Boost Workforce Development
Introduction Performance appraisal has seen a tremendous change over the years. It is no longer just a grading of employees once in a…
