Crowdsourcing digital solutions to master customer data management
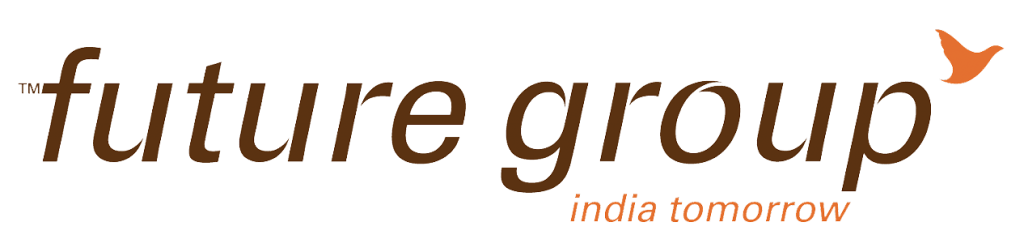
“We came to HackerEarth with a framework on how to become a data-driven organization. We realized the power of using the ecosystem at large to innovate and solve consumer problems. It was a great experience and when we are able implement some of the applications that were built, I believe they will have a far-reaching impact on the business.“
Mr. Vivek Biyani, Director, Future Group
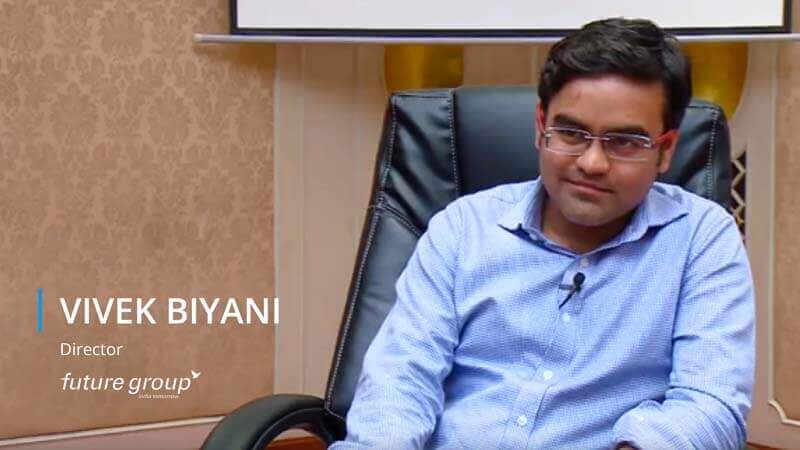
“We came to HackerEarth with a framework on how to become a data-driven organization. We realized the power of using the ecosystem at large to innovate and solve consumer problems. It was a great experience and when we are able implement some of the applications that were built, I believe they will have a far-reaching impact on the business.“
Mr.Vivek Biyani,
– Director, Future Group
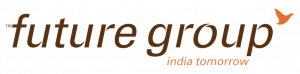
About Future Group
Future Group is one of the largest retailers in India. As one of India’s retail pioneers with multiple retail formats, it connects a diverse and passionate community of Indian buyers, sellers, and businesses. The company employs over 45,000 employees in India and around 400 million people walk into their stores each year and choose products and services supplied by over 30,000 small, medium, and large entrepreneurs and manufacturers across India.
Future Group’s Consumer and Digital (C&D) Labs is an open innovation platform focused on accelerating the realization of Future Group Businesses’ digital strategy. Future C&D Labs chose HackerEarth as its partner to help crowdsource digital solutions to derive meaningful insights from customer data through The Future Datathon 2017. The two-phase hackathon had close to 4500 participants from diverse talent backgrounds who used Machine Learning to build predictive models to understand customer buying behavior and their needs.
The ‘why’ behind the Future Datathon
To deepen customer understanding and strengthen customer loyalty, data can be a goldmine of all customer insights. But most times customer data is scattered and duplicated across multiple systems, making it difficult to access and comprehend valuable insights.
The idea was to mine this vast data to develop rich profiles of households that form the immediate catchment area of a specific Big Bazaar store. Data was collected from seven stores across six cities in India over a period of two years. The business challenge was to identify ways to develop richer, more granular, and a data-led understanding of the households that live in each catchment area around stores. This would then be used to define the clusters of households around each store based on their buying behaviour to aptly predict the products or services required to serve the customer better.
“Future Group has a strong affinity with its customers. We sought to understand our customers better so that we can serve them more effectively. This is a happier experience for our customers and better business for Future Group.“
Mr.Rajiv Prakash, Advisor
– Digital Transformation, Future Group
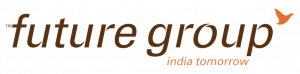
Establishing the groundwork
With over 200,000 data points under consideration, the raw data set was highly complex. This data did not have any natural or surrogate keys present using which one could join files from different databases. It required merging of this information to create a dataset which could have ample data for analysis.
HackerEarth provided its expertise in transforming Future Group’s business problem to a machine learning problem. Furthermore, it helped restructure the data into a decipherable format through in-depth coding and computation. This fine-tuned data was then shared with the participants for data mining and model creation. This allowed the participants to work directly on the problem rather than spend time figuring out the data.
What was the objective of the hackathon?
The Future Datathon brought forth a real-world challenge which when solved could have a direct impact on Future Group’s business. Future Group sought to leverage crowdsourcing and use the vast amount of data at hand to find innovative solutions to business problems.
The main objective of the hackathon was to use machine learning to understand customer behaviour and buying needs better.
There were two main problem statements:
- Clustering problem: Participants had to predict clusters of customers by store locations to predict buying behaviour
- Recommended products problem: Participants had to predict the next 20 products that a customer would buy in the next 1 month
What was the outcome?
4500 Participants
211 Submissions
14 Finalists
3 Ideas chosen
Different approaches to finding solutions were presented by the 14 finalists who brought several innovative models to the table.
“FactorBranded Data Warriors will democratize the thinking of the participants to solve other problems in their own domain or come up with a different solution altogether for a different challenge using the basics that they’ve learnt here. And, that’s the biggest takeaway for the data science community.”
Kanav Anand,
Future Datathon
What were the winning solutions?
The solutions that stood out
Solution 1
The Elbow method was used to determine the number of clusters and the K-means algorithm was used to form clusters based on features such as age, number of products purchased, number of visits, discount and promotional codes used, monthly total and average selling price, etc. Once the clusters were identified, product recommendation was made by predicting buying patterns of each customer using the Apriori method.
Solution 2
Clusters were formed based on the following features using the K-means algorithm :
- Season, temperature, and climate: This gave an idea about which city to cluster during a particular season.
- Religions: This gave insight into the percentage of people belonging to a particular religion.
- Supermarkets, income, literacy and density: This was formed to gain insights and form clusters based on competitor supermarkets, average income of the population, literacy rate, and population density.
Based on the inferences drawn from these clusters, a recommender system was created to recommend products using a recommendation system based on product users.
Solution 3
RFM(Recency, Frequency and Monetary value) analysis was used to predict past purchase behaviour to segment customers. Based on this, customers were segmented into
- Best customers: Customers who bought most recently, most often and spent the most
- Loyal customers: Customers who bought most recently
- Big spenders: Customers who spent the most
- Almost lost and lost customers: Customers who haven’t purchased for some time but have purchased and spent the most in the past
- Lost customers who spend less: Customers who purchased long ago and have spent very less on the purchase
Once the clusters were formed, product recommendations were made using the GraphLab framework.
What was the value derived?
The hackathon helped to add value to the process of data management through the crowdsourcing model. It helped to bring a a new perspective on innovation.
- The hackathon helped Future Group analyse internal data to derive useful insights. Even with up to 200,000 data points under consideration, a lot of observations were gathered from the data.
- It helped Future Group prepare the data better for machine learning challenges in a crowdsourcing model while anonymising and sensitising data keeping in mind the privacy consent and confidentiality requirements.
- Significant value was derived from understanding how to define specific problems for machine learning engineers while maintaining preciseness to derive reasonably accurate solutions instead of abstract ones.
- It brought about diverse ideas not only from an external community but also from internal teams.
Why HackerEarth?
“We were looking for someone who would not only provide a solution but would actually own the business objective and work closely with us as a partner to achieve it. We really liked the amount of ownership and personal commitment that was shown by the team at HackerEarth. They understood the job and had the required expertise to get it done.”
Mr.Rajiv Prakash, Advisor
Digital Transformation, Future Group